Judges and Judiciary,
Technology
Dec. 29, 2022
Machine intelligence in court: bias & transparency
AI is only as good as its training, and it’s tough for users (and the public) to determine the bias resulting from limited training.




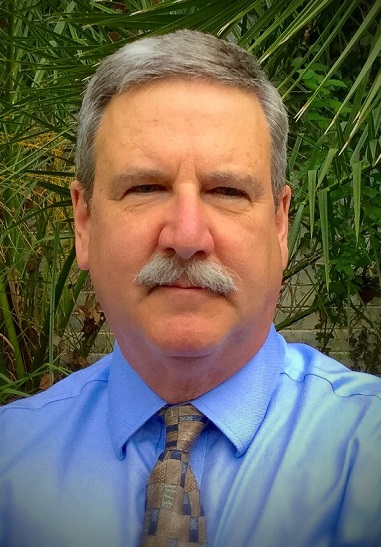
Civic Center Courthouse
Curtis E.A. Karnow
Judge
San Francisco County Superior Court
Trials, Settlements
Judge Karnow is author of "Litigation in Practice" (2017) and current co-author of Weil & Brown et al., "California Practice Guide: Civil Procedure Before Trial" (Rutter).
Artificial intelligence (AI) is used in litigation, e.g., to isolate in large document sets privileged or confidential items. I have suggested (only slightly tongue-in-cheek) its use in providing expert opinion. "The Opinion of Machines," 19 Colum. Sci. & Tech. L. Rev. 136 (2018). And I have reported that Chinese courts are apparently using AI to help decide cases. "Stochastic Gradient Descent," The Daily Journal (Aug. 3, 2022).
I distinguish programs that, despite the hype, aren't AI at all [at least not machine learning]. Some are used to measure the risk of recidivism or failure to appear in court. These are based on studies that show correlations between certain features (e.g. past arrests) and (say) new crimes. The program generates a risk based on these correlates. Few know much about the studies, if their populations resemble the one on which the program is used, if they have been peer-reviewed, or the strength of the correlation. In any event, this isn't machine learning: it's not the product of large amounts of data-generating algorithms, which are in turn used to analyze new data; it's just the mechanical implementation of correlates from studies.
The use of AI to assist or replace judicial determinations implicates many issues - most of them obvious with a few minutes' reflection. They include reliability and accuracy; bias; transparency; human accountability (presidents and governors don't appoint software to the bench); and accessibility.
This note picks out two important issues for AI in courts: bias and transparency.
A central advertisement for AI is that it will reduce bias and augment transparency by expressing the factors that go into legal decisions. So for example, instead of judges deciding on longer sentences because they are hungry just before lunch (as one infamous study suggested), or because the defendant appears to be of a certain ethnic background, AI ignores the irrelevancies. AI is never impatient, doesn't care who the lawyers are, has no passion and no gender bias. It presumably has access to a massive number of like decisions, so it can abstract principles from data no human could traverse in a lifetime. And because we know how it was programmed, we know AI's criteria - which is more transparency than we get from human judges.
So it may be thought.
First, a few words on how true AI - machine learning - works (details are in my Opinion of Machines, Karnow, C. E. (2018). Science and Technology Law Review, 19(1) article). The system is given training data: large amounts of raw input such as documents or animal pictures (or, if we wanted a legal oracle, court opinions). In response to some input, the system generates an output, say "privileged email" or "dog," and if it's wrong, the system's internal state (comprised of "hidden layers") is tweaked until the output is correct. AI now does its own tweaking: it adjusts the very large number of its internal values until it achieves success. Then it's validated on new data.
The internal state of the system, not visible to the human user, is the program. Machine intelligence is a black box; that's why we call its internals 'hidden' layers. No one knows precisely why it succeeds - although in many domains, such as the almost impossible feat of playing world-class Go, folding proteins, spotting credit card fraud, detecting cancer from x-rays, and figuring quantum physics - it is very successful indeed.
There is a new subspecialty known as explainable AI (XAI) to enable trust and transparency, and many large vendors say they provide it. But commentators have noted that XAI can be manipulated and its explanations misleading. Machine learning algorithms are highly complex and often cannot be "explained" to anyone much less an ordinary user; the system and training data are often proprietary and secret; and many systems have not been validated against enough new data to determine if the training was adequate.
So much for transparency.
I turn to bias. Many AI systems are trained on real world data, so they replicate its results--in all of its horror. Some systems recommend hiring men over women, and discriminate in lending against people of color. Some facial recognition software is lousy at distinguishing minorities and especially minority women (the training data used had few examples). Text descriptions of images, as well as image generation software based on text, may associate Muslims with violence, because that bias is found in real world chatter.
Ironically, data can be biased both because (i) it's representative of a biased world, and (ii) contrariwise because it's unrepresentative, such as estimating cardiovascular risk on data from Caucasian (but not African American) patients. AI is only as good as its training, and it's tough for users (and the public) to determine the bias resulting from limited training.
The bias problem remains when AI is used to help or replace human judges: AI may not avoid systemic bias. But AI can rein in idiosyncratic biases: the judge who imposes, relative to other judges, longer or shorter sentences on certain kinds of people; or a judge always grants (or denies) certain kinds of motions, e.g., class certification, summary judgment, sanctions - in short, AI can (in theory) forestall arbitrary decisions, and maybe other outliers.
But AI can do this only if it has enough training data for pattern detection. And the greater the variability of the data the more of it we need, just as statistical analyses require larger samples as variability increases. Duran v. U.S. Bank National Assn., 59 Cal.4th 1, 42 (2014).
How variable is judicial decision-making? The outcome of a motion may be binary (granted or denied) suggesting low variability, but in truth most of what judges do is extremely variable. Consider factors in summary judgments: countless evidentiary rulings, legal analyses (differing case to case), reviews of whether scores of allegedly undisputed facts are supported or opposed by evidence. No one argues that a demurrer in, say, a malpractice case for just that reason be decided the same way as some other malpractice case. We recognize the many ways in which cases in the same area and at the same procedural step materially differ.
So having enough training data to enable generalization is problematic. But without it, AI is biased. We may have data for a few isolated, relatively simple domains. Indeed, A.I.'s successes have been in tightly delimited domains, but for the foreseeable future that still leaves pretty much all of the judicial work to humans.
We don't have to digitize our black robes quite yet.
This is the final article in a series of columns focusing on how artificial intelligence is impacting attorneys in and out of the courtroom.
Submit your own column for publication to Diana Bosetti
For reprint rights or to order a copy of your photo:
Email
Jeremy_Ellis@dailyjournal.com
for prices.
Direct dial: 213-229-5424
Send a letter to the editor:
Email: letters@dailyjournal.com